Trying To Augment Intelligence With AI Fails When Data Scientists And Designers Don’t Collaborate
Augmenting human intelligence is the fastest way to get value from AI. The problem? Human-centered design (HCD) is missing from most attempts to augment human intelligence.
Sure, the AI teams doing this work sincerely care about humans — but that’s not the same thing as knowing and applying proven HCD methods like iterative prototyping paired with disciplined observation of users. Think about it this way: What if designers believed they could produce rock-solid code because they care about quality — not recognizing that software quality requires proven software engineering methods?
But don’t just trust Forrester’s conclusions about this — examine the evidence:
Research from Carnegie Mellon reports: Despite success in labs, the vast majority of clinician-facing decision support tools failed when moving to clinical practice; researchers identified a lack of HCI consideration, rather than poor technical performance, as the main cause for these failures. (HCI means human-computer interaction.)
A researcher at Stanford points out it’s a problem in the legal world, too. Legal professionals ask: “Why should they follow the recommendations of a model built by a company that they know nothing about, using data they do not control?” The issue here isn’t the source of the data; it’s that somewhere along the way, users’ functional and emotional goals aren’t being met.
There are many other examples out there. When we spoke with argodesign founder Mark Rolston, he pointed out that this kind of augmentation is one of the most interesting design challenges today. He asked, “How do you educate users that the machine might be wrong?” His perspective: “If the interface is constructed right, being wrong is OK.” We agree, because getting the user interface right means ensuring that users know when a computer is simply presenting existing information as opposed to recommendations that are speculative because they’re based on an educated guess derived from detecting patterns in data.
argodesign tackled this in the Sano wearable — a device focused on glucose monitoring for diabetics that gives them guidance and recommendations, with helpful markers in this spirit. The app includes dotted lines to indicate predicted blood sugar, solid lines to show previous days, a confidence score, and recommended corrective actions (see image below). argodesign isn’t the only design provider working on this challenge — other services companies have recognized the need and are creating offerings focused on designing for augmented intelligence.
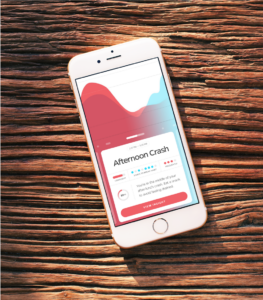
For more examples and several conceptual frameworks for getting this right — such as the distinction between agentive and assistive technologies — see my new report: “Data-Fueled Products: How To Thrive On The Design And Data Science Collision.”
If you’re working on these challenges or have questions, get in touch — I’d love to hear from you!